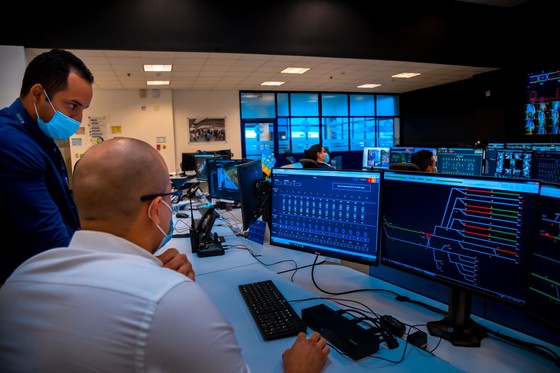
How data science is becoming a game changer in the mobility industry
How data science is becoming a game changer in the mobility industry
Better vehicle utilisation, more efficiency, less delays and overall, higher service quality and customer satisfaction – the ongoing demand for a more comfortable transport experience can put an enormous amount of pressure on mobility operations and maintenance, resulting in complex digital systems. In order to handle this expansive digital infrastructure, it’s necessary to collect, analyse and utilise huge amounts of data. In other words, data science is becoming, more and more, a game changer in the whole mobility sector and Alstom is at the forefront of this field.
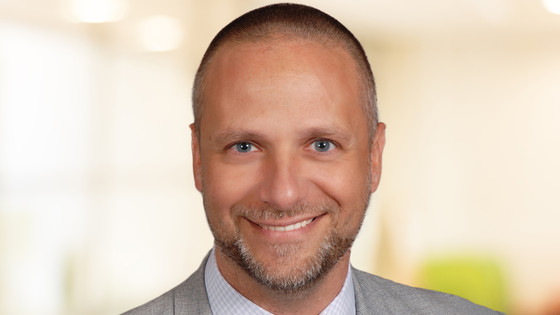
Before joining Alstom, Nenad has held several R&D and leadership positions in startups, blue-chip companies and as a researcher and a lecturer. He has over 20 years of experience in algorithm development, machine learning and AI. Nenad started his journey with Alstom as a Senior Data Scientist in Melbourne, FL, USA. In his current position based in Saint Ouen, France, Nenad leads Alstom's data science teams responsible for delivering Machine Learning and AI algorithms for all mobility solutions.
In his spare time, Nenad enjoys art, reads science fiction, philosophical and historical books, and plays tennis. Last but not least, he evangelises math and computer science skills to girls and young women, be it in his own family or in schools and public events, encouraging them to pursue technical degrees.
Connect with Nenad on LinkedIn
“Data science is one of the main pillars of Alstom's digital strategy”, says Alstom’s Data Science Leader, Nenad Mijatovic. He and his team are responsible for developing advanced statistical and machine learning solutions which support maintenance and operations decisions. “We use data as input and create business insights as output," says Nenad Mijatovic. He is convinced that data science is becoming the main tool to solve existing problems and create new business cases within the whole industry.
One concrete example is the optimisation of odometry processes: Train protection systems rely on the estimated train location data from the onboard odometry and signalling systems. Odometry event anomalies could trigger manual train operations or, in some cases, even train stoppages. System experts must review terabytes of onboard data, collected across the fleet, to spot event anomalies and prevent these situations. “This is a highly time-consuming process which can be substituted by advanced algorithms and data analytics”, explains Nenad Mijatovic.
“For the first time, our platform leaders, maintainers, and domain experts are equipped with solutions that allow them to process a large amount of data and automatically detect problems, classify issues, and propose actions.”
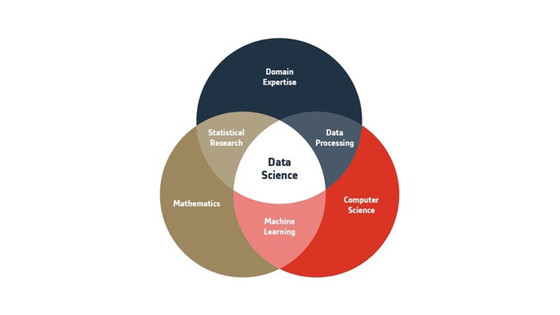
4 key solutions to data science challenges
- Alstom created a dedicated group of data-driven professionals through educational workshops.
- The quality of data was improved by developing a methodology and software that allowed only ‘good’ data to reach inputs of Alstom’s data science algorithms.
- Alstom developed a data science methodology and technology readiness levels way of working to ensure a ‘zero-to-hero’ result.
- The machine learning operations paradigm was adopted and adjusted to support the full life cycle of models.
What is special about Alstom’s approach?
“Alstom's approach to data science is inclusive thus differing from that of most other mobility companies”, says Nenad Mijatovic. Using a highly collaborative environment with domain experts and customers, the data science team first understands the problem at hand and then develops a model using different science techniques. “We iteratively converge to the best model before we put it in production. Finally, we closely monitor model performance in production to make sure it performs within given performance boundaries”, explains Nenad Mijatovic.
The benefits of this approach are obvious: data science supports better maintenance by applying condition monitoring and predictive analytics; faster troubleshooting by applying a smart root cause analysis; efficient operations by applying simulations, digital twin, and reinforcement learning techniques; and an improved transport experience of the customers by detecting degraded or faulty rolling stock or track equipment.
“Through a highly collaborative environment with our domain experts and customers, we first understand the problem at hand, monitor and then develop a model using different science techniques, and iteratively converge to the best model before we put it in production”.
The 3 main components of Data Science at Alstom
How data science can push sustainable innovations
Alstom's expertise in data science is a key benefit in the industry: Recently, Alstom has announced a contract with the sustainable innovation laboratory of MerConcept to optimise their data analysis by using data science for sustainable mobility in challenging environmental conditions. The partnership includes a deep dive data analysis followed by the development of the optimisation algorithms to improve the speed of offshore racing boats in a competitive environment. Created in 2006 by the sailor François Gabart, MerConcept’s mission is to elevate the progress made in sustainability in sailing and to contribute to showcasing the innovations and the advanced technologies used in offshore racing today.
“To decrease the carbon footprint, we support optimal rolling stock operations. To reduce waste disposal, we reduce the number of repairs. To address climate change, we alarm the maintenance about infrastructure issues to prevent incidents.”
What comes next?
Current data science technologies and tools are well designed and matured to predict the future based on the past. “However, for many problems, we do not have much of the quality data points, based on which we can develop reliable methods”, says Nenad Mijatovic. “Thus, I think that the future for us as data scientists at Alstom is to research new methods which can work on sparse data points, or in some cases, no data points of interest.”
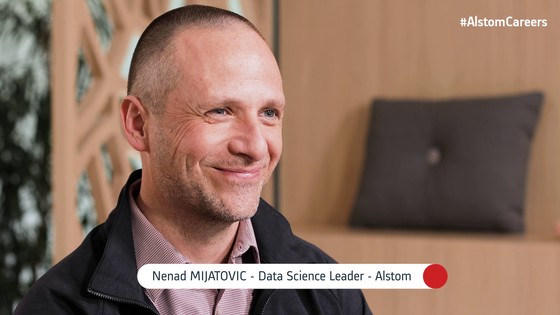
Interested in joining our data science teams? We have many opportunities available worldwide! Get to know Nenad in this video and make sure to browse for our open positions using the link below.